For the last year, Anna Sroginis and I have been working on a paper, trying to modernise demand classification schemes and make them useful in the brave new era of machine learning. We have finally wrapped it up and submitted it to a peer-reviewed journal. But the temptation to share was too strong, so we […]
Svetunkov & Sroginis (2025) – Model Based Demand Classification
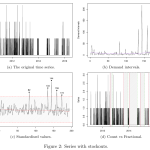