Here is one thing you have probably not heard of: a workshop on Open Source Forecasting software, held in Beijing on 26th – 27th June 2025. This was a closed event, with speakers attending by invitation only. It focused on recent advancements and potential avenues in open-source forecasting software. But why am I writing about […]
IIF Open Source Forecasting software workshop and smooth
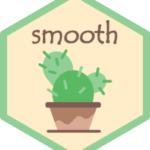